Each year, the FDA receives over two million reports of suspected deaths, serious injuries, and malfunctions associated with medical device malfunctions. As the range of medical equipment continues to rapidly increase, healthcare facilities are becoming even more dependent on the consistent and uninterrupted performance of their devices. Any unexpected failure of medical equipment can lead to significant financial losses and, even worse, cause harm to patients.
One of the efficient ways to deal with possible breakdowns is to prevent them before they cause irreversible damage. That's where predictive maintenance comes into play.
In this article, you’ll find out what predictive maintenance is, how it differs from a preventive approach, and what it takes to implement it.
Medical device maintenance before predictive analytics
For many years, healthcare facilities have relied on preventive medical device maintenance – regular in-person equipment inspections.
Usually, maintenance teams conducted their assessment according to a predetermined schedule. Even though routine inspections could prevent potential breakdowns in advance, they were sometimes inexcusably late, as unexpected device failures could happen when the next inspection was still far down the calendar.
Unpredictable incidents were often one of the culprits of hospital disruptions, resulting in wasted resources and delays in patient care. Moreover, since preventive maintenance of medical equipment occurred on schedule, sometimes the maintenance team checked the equipment even if there was no rational need for that, which led to unnecessary work and increased operational costs.
However, the rapid development of AI, ML, and data analytics introduces a more efficient way of maintaining equipment.
Predictive maintenance appears as an alternative to traditional maintenance. It relies on advanced monitoring tools, like IoT sensors, AI, and ML algorithms, to analyze the current state of the medical asset. If there is any anomaly in the device's work, the algorithms trigger an alert, notifying the maintenance team to respond proactively. This way, the maintenance team can plan the procedure of device repair beforehand without disrupting the hospital's flow.
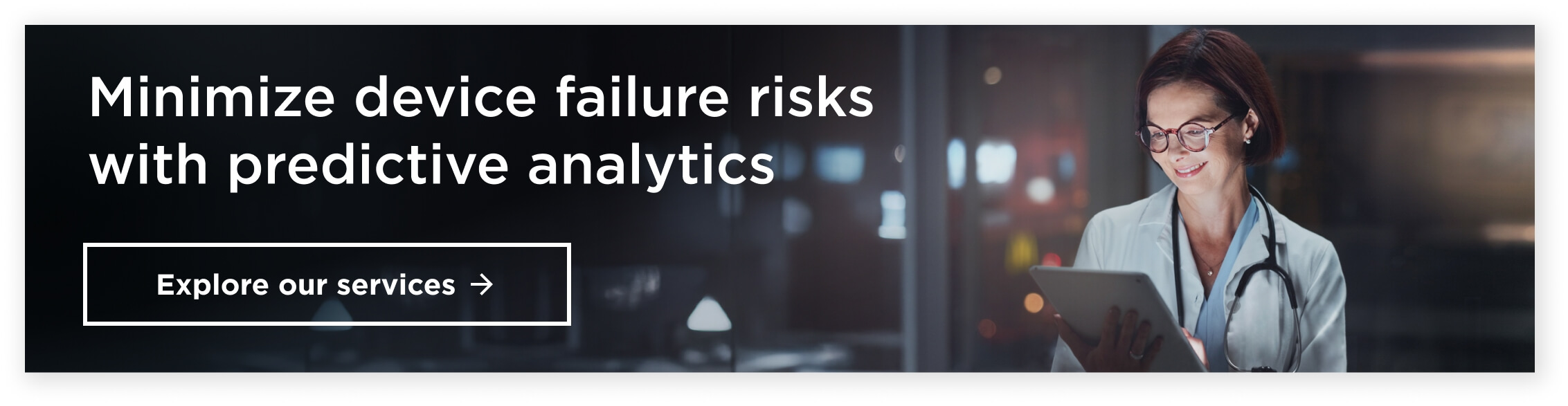
The comparison table below shows the differences between traditional and preventive maintenance approaches.
Preventive maintenance | Predictive maintenance | |
---|---|---|
Approach | Scheduled with fixed intervals | Proactive (before the failure of the device happens) and based on real-time data analytics |
Device downtime | Unexpected failures and equipment downtime can occur before scheduled inspections | Planned and controlled, executed during non-peak times in the hospital |
Safety risks | Higher, as failures can appear unexpectedly anytime, endangering patients’ lives. | Lower, as the system warns about possible malfunction, so that some actions can be taken in advance |
Cost efficiency | Doesn’t require high upfront costs, but it is less cost-effective as maintenance is performed regardless of the actual condition of the device | Demands higher upfront costs to incorporate the data analytics solution, but in the long run is cost-effective as maintenance is carried out only when needed |
Implementation | Simple to implement, as it requires basic monitoring assets and experts with basic knowledge of medical device maintenance | More complex to implement as demands advanced monitoring tools and data analytics experts |
How does predictive maintenance work?
Predictive maintenance works based on several components:
- Condition-monitoring sensors
- IoT technology
- AI-based predictive algorithms
- Data visualization platform
Below, we unpack each of the components, illuminating their key functions that make predictive maintenance work efficiently:
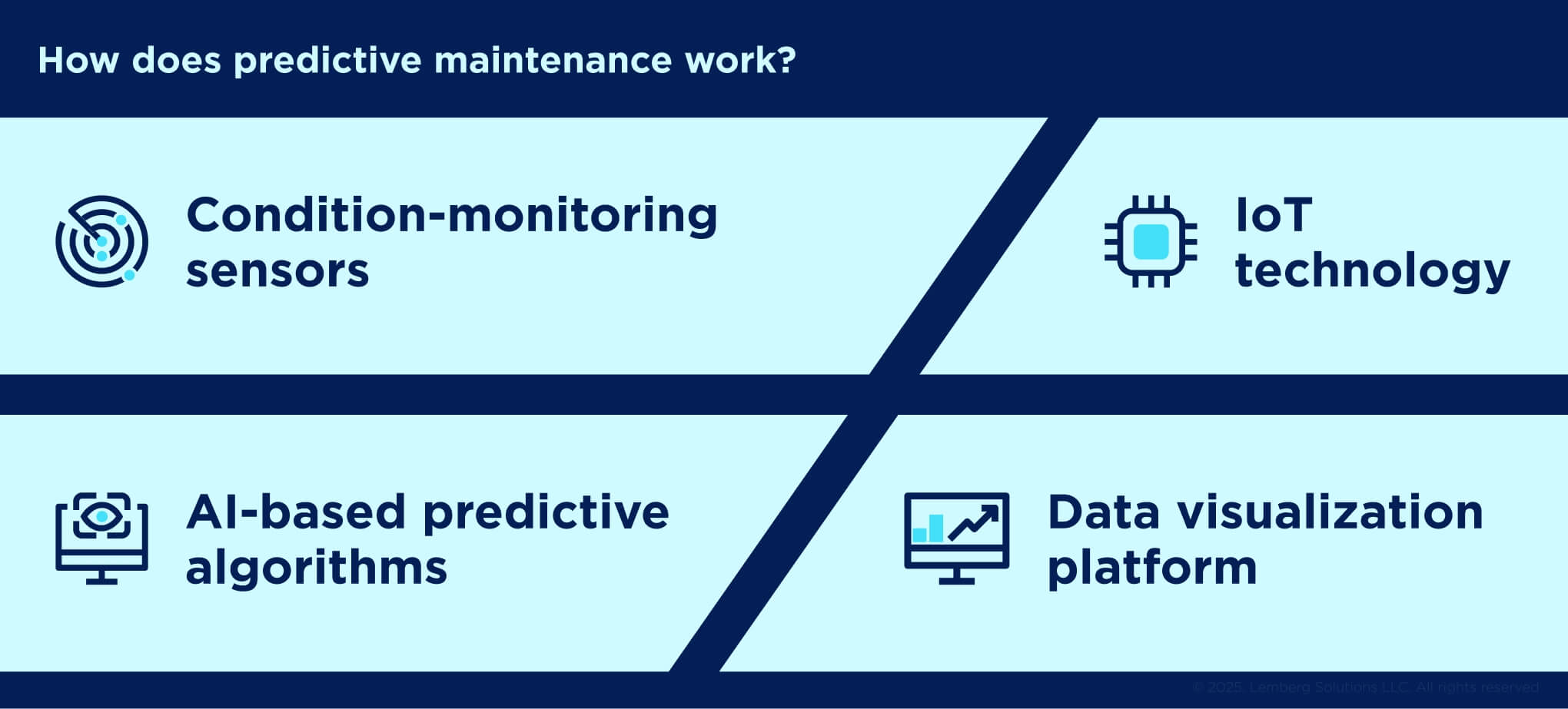
Condition-monitoring sensors
Predictive maintenance begins with gathering data from the medical device with the help of embedded sensors. They are the eyes and ears of the maintenance team, continuously collecting data from equipment, tracking different critical metrics like temperature, vibration, current, or even image quality (for example, in computer tomography). Capturing various metrics in real-time, these sensors provide more detailed insights into the device’s health.
IoT technology
Once sensors are embedded in medical devices, the next important task is to ensure that the data they collect is delivered to the right place for analysis. That’s where IoT connectivity comes in, making sure that data is correctly transmitted to the cloud platform for predictive analytics. The cloud platform provides the storage needed to securely process vast amounts of data. However, with increased connectivity of devices, so does increase the responsibility for ensuring the security of the IoT connection.
Read more about key IoT-related risks in healthcare and practical solutions for avoiding them in our article.
AI-based predictive algorithms
AI-based algorithms are the ones that do all the "magic" of the predictive analytics process. They analyze both historical and real-time data to understand how a device works in a normal state and to detect early signs of any anomalies in its behavior. For example, an MRI machine has sensors that monitor temperature, magnetic field, image quality, pressure, and sound. By continuously analyzing the collected data, AI algorithms can identify even subtle changes in these metrics, alerting maintenance staff to fix problems before they lead to device failure.
Data visualization platform
An essential part of the predictive maintenance system is the human-machine interface. Data visualization platform transforms complex datasets into actionable insights, presenting them in a user-friendly manner. This platform provides the maintenance team with visual reports, graphs, and alerts, enabling them to assess the equipment's health state at the moment and track the performance patterns over time to detect potential anomalies.
Key benefits of predictive maintenance for medical devices
While the adoption of predictive maintenance in the healthcare sector still moves slowly, nevertheless, its potential to transform the maintenance of medical equipment is undeniable. See below the list of key benefits that make predictive maintenance worth pursuing:
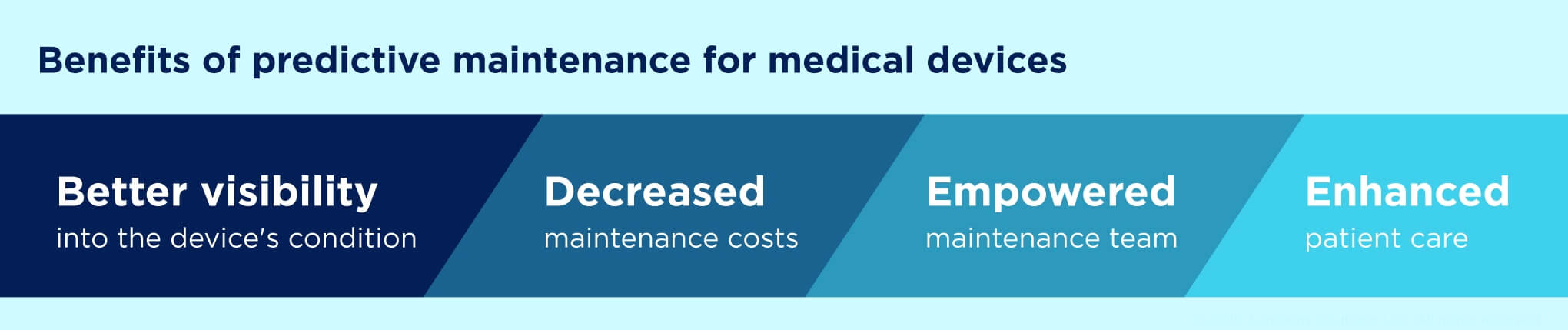
Better visibility into the device's condition. The continuous monitoring of the medical asset with sensors allows AI algorithms to provide detailed analytics of the device's current state. Overseeing the changes in performance and fixing them as soon as possible helps to slow down the degradation of the equipment.
Decreased maintenance costs. Predictive analytics minimizes unnecessary scheduled maintenance, ensuring interventions are made only when truly needed. Such an approach allows for planning the equipment repairs, therefore reducing the possibility of costly emergency repairs and maintenance, resulting in significant savings.
Empowered maintenance team. Equipped with real-time data analytics, asset operators and service providers shift away from reactive troubleshooting to more proactive equipment maintenance. With insight into the device’s state of health, staff can efficiently plan maintenance activities and allocate resources
- Enhanced patient care. The proactive approach of predictive maintenance allows to reduce the risk of machine failure during patient treatment. Therefore, healthcare providers can deliver high-quality care to their patients, without delays in diagnosis or treatment and without risking patient safety due to unexpected device failure.
How to set up predictive maintenance for medical devices?
Now, that you know the key components and benefits of preventive maintenance, the next logical question is where to start. Here are the essential steps to follow:
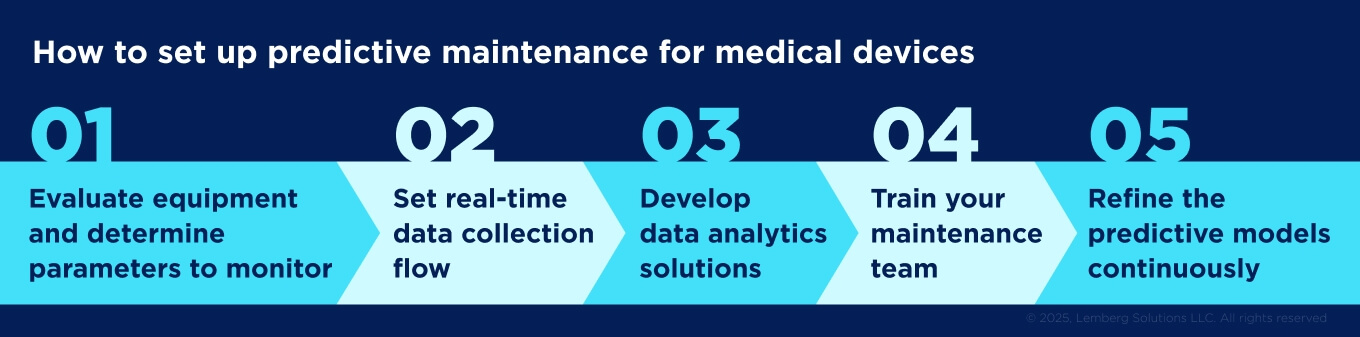
#1 Evaluate equipment and determine parameters to monitor First, start by assessing medical equipment to understand its current state. Identify which existing medical devices will benefit most from predictive maintenance, specifically considering those critical for patient care and with high repair costs.
#2 Set real-time data collection flow
No predictive maintenance is possible without consistent data flow – so the next crucial step is to set up a real-time data collection pipeline. It is better to ensure this task is entrusted to the experts who know how to organize tools to handle data collection, adhering to all security measures. A team of professionals will manage the incorporation of built-in or external data collection mechanisms into your medical devices for proper gathering of the data according to specific parameters.
#3 Develop data analytics solutions
Once you've set up your data collection pipeline, the next step is to work on the AI and machine learning-based data analytics solution with a team of data and AI experts who will help you design and deploy it. Experts will prepare and test the analytics solution using both historical and real-time data to tailor it to the specific needs of your healthcare organization.
Artificial intelligence and machine learning algorithms detect early signs of anomalies in the device’s workflow. When there is a clear understanding of when a problem might occur, the maintenance team can take the necessary steps in advance by creating a plan for repair or purchasing new equipment.
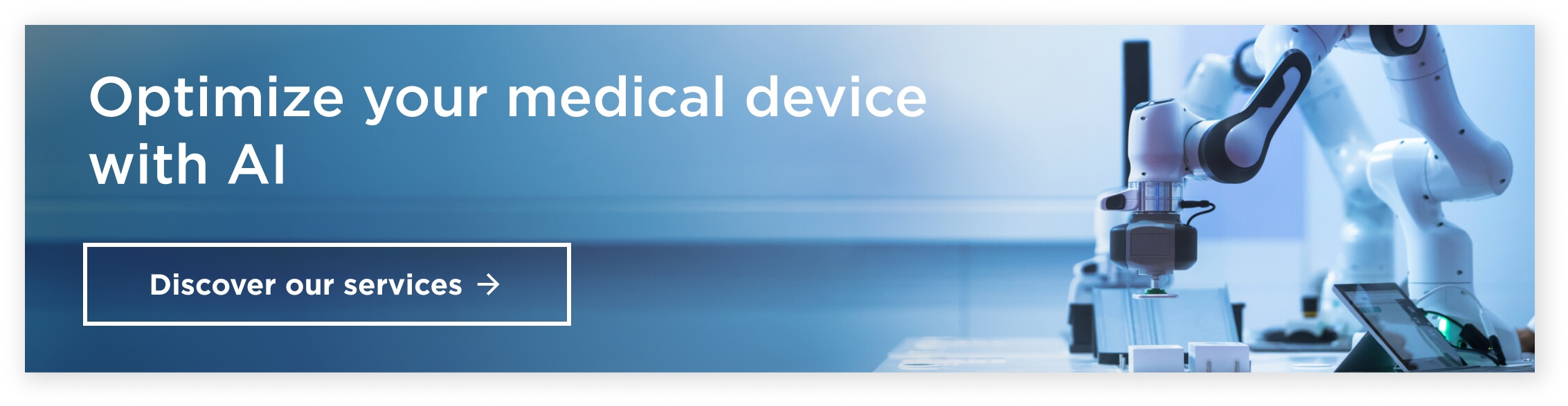
#4 Train your maintenance team
After the predictive maintenance solution is incorporated into your healthcare system, the next step is to ensure your maintenance team understands how the new technology functions. Staff training should cover operating the sensors, interpreting the data, and responding to predictive model warnings. You should create a standardized procedure and define roles and responsibilities between your maintenance team to ensure reliable and quick action to prevent device downtime and minimize costly unexpected repairs.
#5 Refine the predictive models continuously
Just because the predictive maintenance system is in place, it doesn't mean that work is done. Regular assessment and refinement of your predictive system are key to keeping it accurate and reliable.
How can Lemberg Solutions help with predictive maintenance?
With over 15 years of proven expertise, Lemberg Solution is a trusted partner in providing top-tier data analytics and AI services in the healthcare domain. Focused on our client's specific business needs, we deliver healthcare software solutions that adhere to key industry compliances and regulations like HIPAA, DiGA, MDR EU, ISO 13485, and IEC 62304.
Our team includes P.H.D.- level experts who will work with your medical data at every stage of developing a predictive maintenance solution – from integrating sensors into your equipment, ensuring IoT connectivity, and setting up an efficient data collection process to developing artificial intelligence algorithms for predictive analytics.
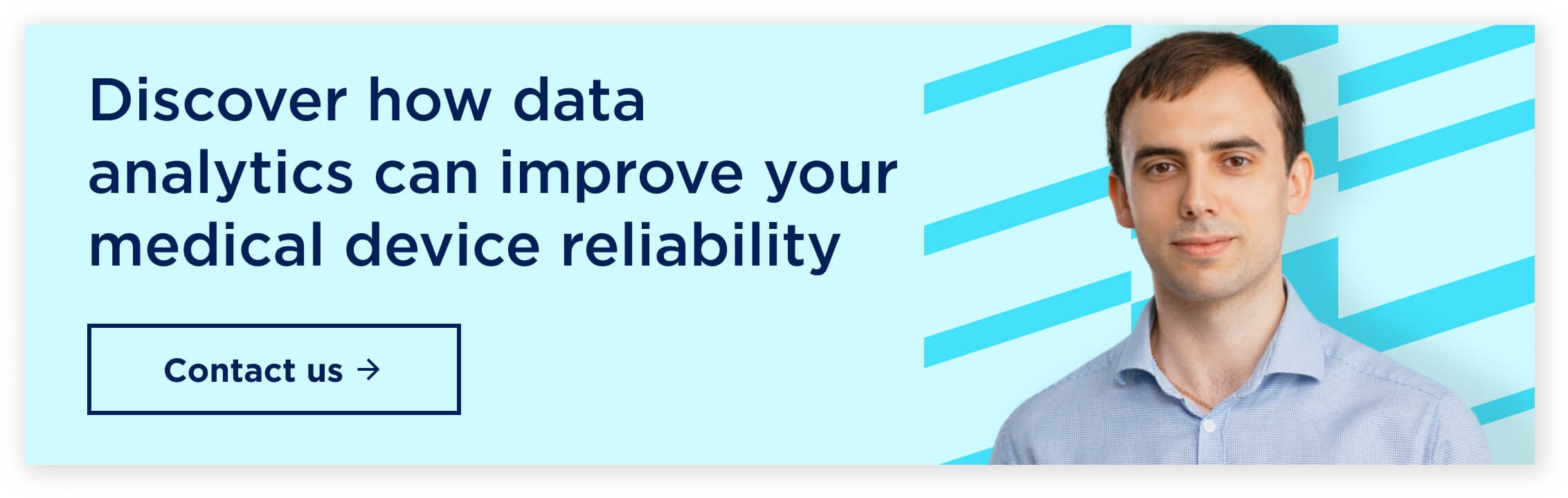
FAQ
What are the types of maintenance for medical devices?
There are three main approaches to medical device maintenance: reactive, preventive, and predictive. Reactive maintenance is one of the oldest forms of maintenance, which is performed only when the device has already broken down or is not operating properly. This approach is considered as inefficient as it leads to unplanned device downtime, high costs of emergency repair service, and therefore a lower quality of patient care.
On the other hand, preventive maintenance allows the maintenance team to prevent equipment failure by conducting regular scheduled maintenance checkups and servicing, which can help identify problems before the device breaks down.
The most recent and proven to be effective way to medical device maintenance is predictive maintenance. Predictive maintenance relies on technological advancements such as IoT, AI, and machine learning. IoT sensors collect data continuously, monitoring the state of health of medical equipment. This data is analyzed by smart AI algorithms, which accurately predict when the asset is likely to fail and plan the optimal time for maintenance.
Why is predictive maintenance important in healthcare?
Predictive maintenance allows healthcare providers to ensure the flawless performance of their critical devices, providing high-quality patient care. Predictive maintenance reduces the risks of unexpected device faults and emergency repairs by addressing any issue before it can disrupt the operational efficiency of the hospital.
What are the benefits of predictive maintenance in hospitals?
Predictive maintenance for medical devices in hospitals brings a variety of benefits. The most crucial one is the safety of patients. By identifying potential faults early on, hospitals can be sure that their critical assets will stay safe and functional with no unexpected breakdown. Also, predictive maintenance optimizes maintenance-related costs by minimizing unplanned emergency fixes and extending the lifespan of assets.
How does predictive maintenance differ from preventive maintenance?
Preventive maintenance follows a time-based approach. It means that medical assets are inspected based on the fixed schedule, regardless of their condition. Even though this approach can be effective, most of the time, it leads to unnecessary maintenance or missed signs of device failure.
Predictive maintenance, on the other hand, follows a condition-based approach. It leverages real-time data collected from sensors and sends it to AI and machine learning algorithms for analysis of the current condition of a medical asset. The maintenance team performs the asset repair only when a potential problem is detected, and such an approach minimizes the wasting of resources on unnecessary checks.