The demand for predictive maintenance (PdM) solutions in the energy sector is rapidly evolving. The global PdM market is projected to grow 31% from 2024 to 2029.
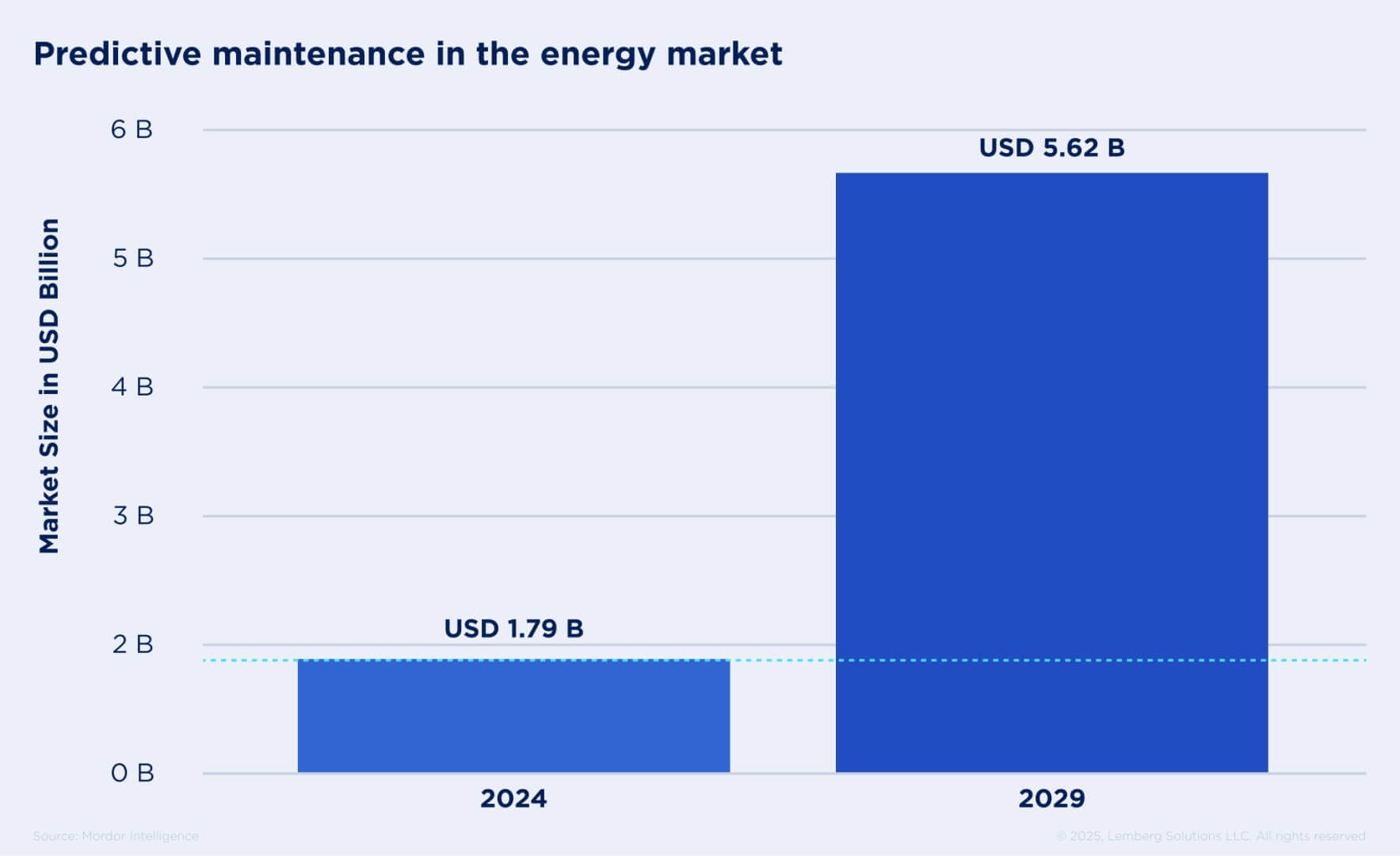
Businesses are willing to protect their critical equipment and prevent downtime; meanwhile, they don't want to waste extra costs on useless repairs and improvements.
In this article, we investigate how predictive maintenance helps optimize the productivity of energy systems while predicting failures, minimizing downtime, and decreasing costs.
What is predictive maintenance in energy?
Predictive maintenance is a strategic approach to monitoring the energy system equipment operation under normal daily conditions to detect potential and actual abnormalities and issues. The main goal of predictive maintenance is to fix any system malfunctions before they cause a severe system failure.
Unlike manual system check-ups, predictive maintenance algorithms combine various data collection tools and analysis techniques to reduce the probability of a system breakdown. Though it might sound too good to be true — with predictive maintenance, you minimize your experts' work hours while also minimizing risks and system downtime.
Why is predictive maintenance worth considering and implementing into energy-based systems? Keep reading to uncover the main benefits of predictive maintenance in energy, as well as its techniques and examples.
Why do you need predictive maintenance for your energy systems?
Predictive maintenance prevails for energy systems, among other ways to maintain them, but why?
For example, corrective maintenance detects system errors de facto, causing emergent repairs and durable downtime. At the same time, preventive maintenance entails scheduled activities that demand extra costs and resources but are not always necessary to keep the system resilient and steadily operating.
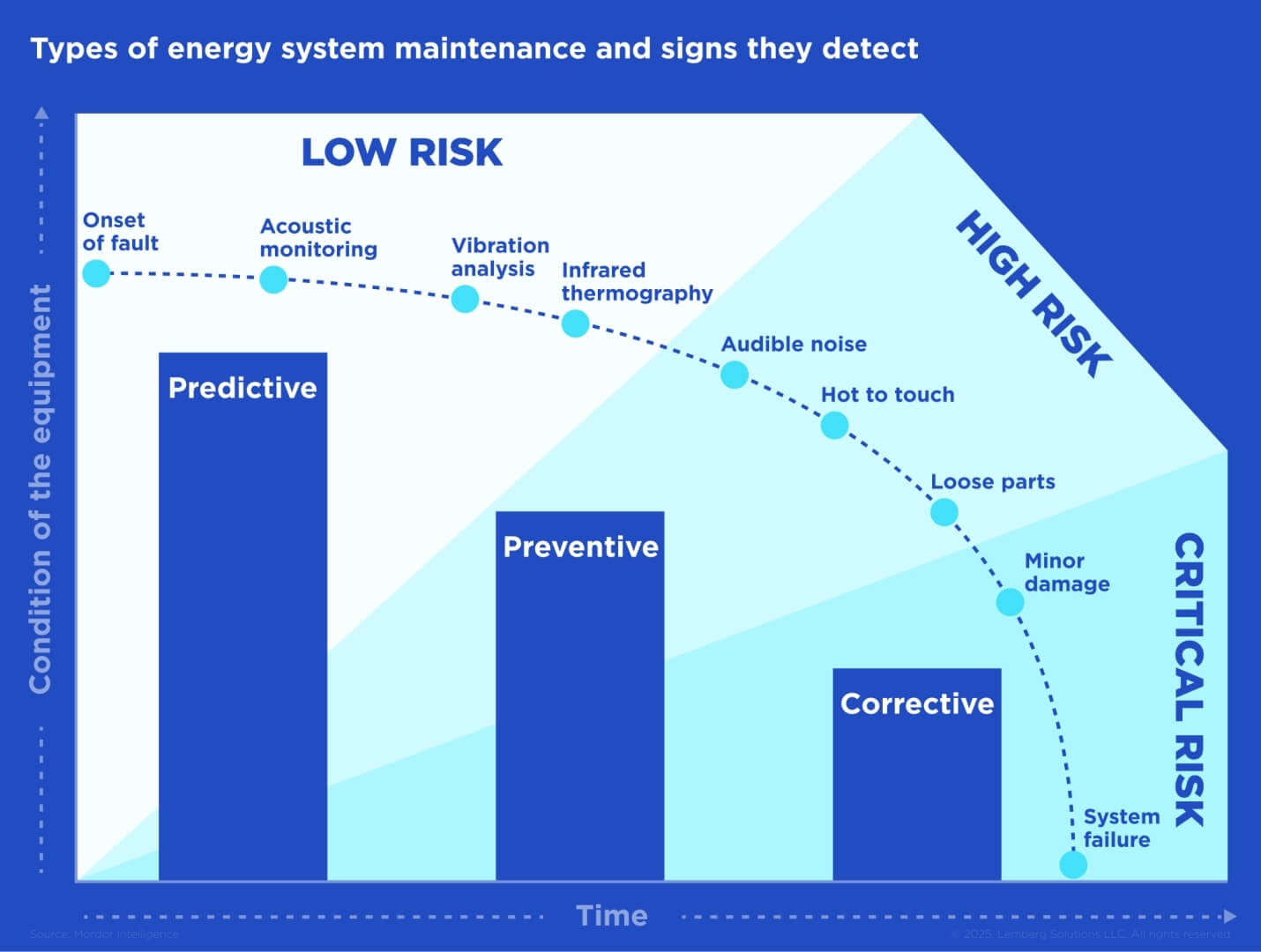
Unlike the previous options, the PdM technique combines constant condition monitoring, collecting and analyzing data from the field to reveal patterns of normal and abnormal energy system operation.
Since predictive maintenance helps optimize energy system maintenance activities, targeting them where needed, it also helps reduce extra costs in the long perspective. Though establishing predictive maintenance requires initial investments, it'll eventually pay off with efficient failure prediction, efficient utilization of resources, and lower operational costs.
How do you save costs with predictive maintenance?
Whatever the type of energy system you work with, predictive maintenance will help you prevent equipment downtime and failure.
Still hesitating whether predictive maintenance is worth trying? Further, you will discover its core benefits for your business and dispel your worries.
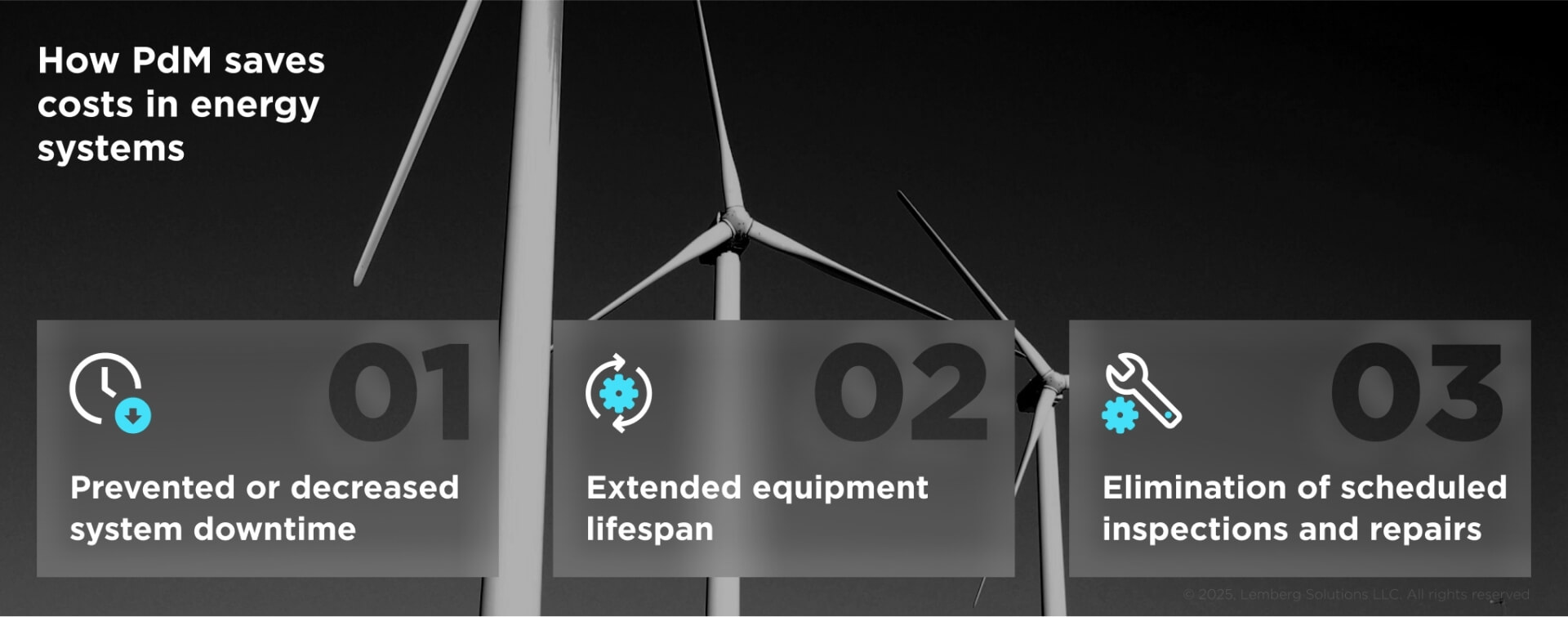
- Prevented or decreased system downtime
Untimely repairs of energy systems lead to durable downtimes, which are enormously costly for businesses for two main reasons – power generation slowdown and a system relaunch that requires massive energy consumption.
Each minute of system downtime costs your company a lot, from spending money on reactive repairs to losing your income, customers, and even the brand's image.
Indeed, the amount of money you will lose depends on the specifics of your business and the production capacity of your energy system. It can vary from hundreds to thousands of dollars. Moreover, such failures can become your frequent guests.
Following the predictive maintenance approach minimizes system failure risks, ensuring the system's smooth operation and timely fixes.
- Extended equipment lifespan
Regular monitoring and on-time replacement of obsolete or broken hardware components extend energy equipment lifespan.
Predictive maintenance defines the right time to repair or replace some components to prevent system failure and prolong asset lifespan, be it wind turbines, solar panels, hydroelectric plants, or other types of energy assets.
At this point, you might be thinking — "Well, do I really need all those repairs and component replacement?" But remember, purchasing and setting up new equipment will cost you significantly more.
- Elimination of scheduled inspections and repairs
Unlike corrective or preventive maintenance, predictive maintenance makes scheduled inspections and repairs unnecessary. The number of inspections of equipment conditions and working environments can be limited to mandatory check-ups.
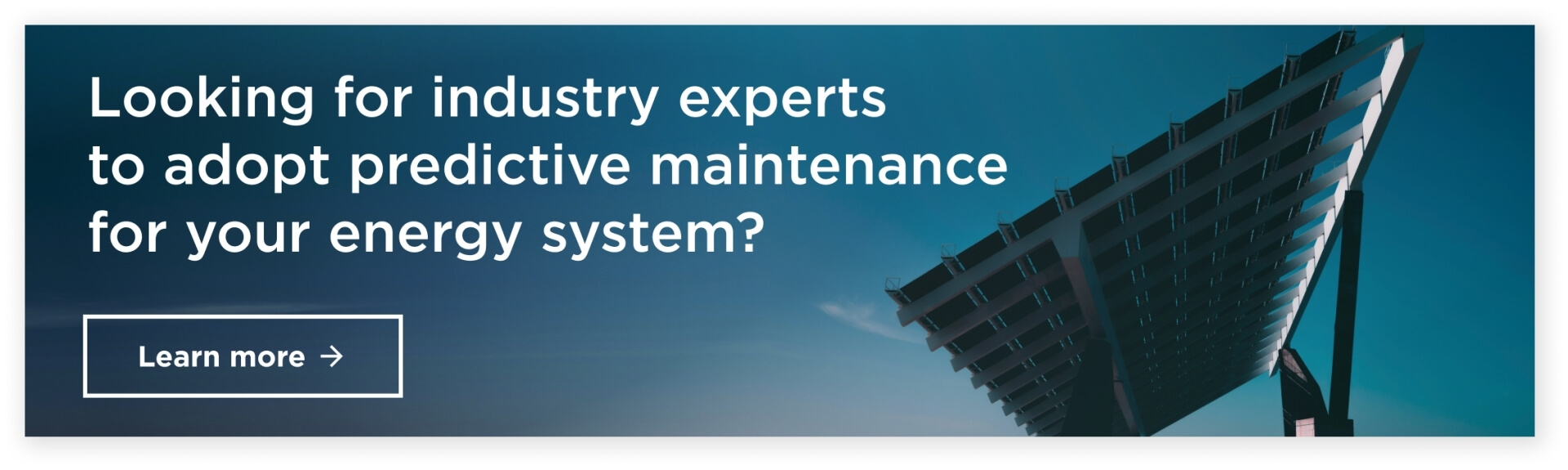
Tools and use cases of predictive maintenance in energy systems
We compiled the most popular and efficient techniques to establish a prediction for your energy system's performance, including the state of health of your hardware.
You might be familiar with some of these tools and predictive maintenance use cases in energy, but they remain a guide to quality system maintenance and cutting excessive costs.
Sensors integration
Sensors are commonly used for condition-based monitoring. They collect real-time data about energy assets' operation and the equipment environment to detect abnormalities early on, fix them, and decrease system downtime. Different sensors can measure temperature, current, vibration, and other parameters you need to track for your system's uninterrupted performance.
For example, real-time monitoring of hydropower plants using sensors allows for collecting the rate, vibration, and water flow data directly from turbines. Then, this data is transmitted to the storage for further analysis on what predictive activities should be taken to avoid malfunction.
IoT connectivity
IoT connectivity ensures real-time sensor data collection from your remote energy assets for further analysis. Sensors collect data, while IoT gateways help transmit it to the local or cloud-based storage in real time to track the equipment performance and reveal any deviations as early as possible.
IoT for predictive energy system maintenance focuses on permanent monitoring and alerting about any issues immediately based on the collected data about vibrations, temperature, noise spectrum, and asset state of health.
Machine learning algorithms
Machine learning is another approach to effectively maintaining energy systems. But how does it work? It’s simple like this — AI-based algorithms analyze the previously stored data to determine the patterns of normal system operation and detect the first signs of abnormal behavior.
For instance, AI algorithms are now commonly used for wind turbines, collecting vibration data from different system components and revealing patterns on normal and abnormal vibration features. It helps fix failures before they occur and properly plan maintenance activities only when necessary.
Discover more use cases of ML-based predictive maintenance by following the link.
Data analytics and visualization
A data analytics and visualization platform helps gain insights into data collected from various sources, including real-time sensor data, operational metrics, environmental conditions, and historical data recorded and analyzed from previous periods.
Data analysis includes various tools and techniques, such as predictive analytics, machine learning algorithms, statistics, and interconnections to build precise predictions on energy system maintenance activities.
How to set up predictive maintenance for your energy solution: 5 steps
You decided to adopt predictive maintenance for your energy system. Where should you start? Let's break down this process into simple steps.
- Step 1: Define the issues you want to fix
The first and foremost step is to determine what issues you want to resolve; for example, forecast equipment failure before it harms your system. Having a specific goal from the start allows you to further define the parameters you need to track to make timely checkups and repairs.
- Step 2: Determine what parameters to monitor
The energy system metrics you need to track vary depending on its purpose and specifics. It may include collecting data on temperature, humidity, vibration, pressure, water flow, etc.
Even more important is to define the critical equipment and its normal performance parameters. Then, you can use historical data to detect abnormal patterns and potential failures as soon as possible.
- Step 3: Establish real-time data collection
After you have defined the type of information you need to receive from your energy equipment, the next vital step is establishing a proper real-time data collection and processing pipeline.
This process includes integrating and calibrating sensors into energy devices, enabling IoT connectivity between hardware and storage. It is crucial to configure existing and newly deployed sensors to collect accurate and reliable data about system operation so that they react to abnormalities as fast as possible.
Gathering quality data allows you to analyze system performance patterns in typical conditions, detect potential issues, and prevent downtime with timely maintenance.
- Step 4: Deploy data analysis tool
Once your data processing is set up and you receive clear and accurate data from your energy devices, the next step is data analysis.
You need to engage data and AI experts to build and deploy ML-powered models using real-time and historical data on stable energy system operations and failures to train and test the models.
After that, the models may need some improvements to adjust to your system`s specific conditions. That's how you get predictions on what failures to expect and will be able to fix them right on time.
- Step 5: Deployment and continuous monitoring
When the ML models have passed training and first tests, the final step is their implementation all over your energy system. Training your maintenance team members on reading ML-based insights and converting them into predictive activities should also be the next step.
At this point, continuous monitoring and improvement becomes an uninterrupted process to optimize your system performance and energy usage.
How Lemberg Solutions can help with implementing predictive maintenance and saving costs
Lemberg Solutions is a reliable provider of data and AI engineering services. Our company gained deep expertise and experience in data collection and engineering, data science, predictive analytics, and AI solution development by delivering projects in energy, healthcare, automotive, and other domains.
Our experts know how to turn your energy system data into a valuable asset for your business, predicting system failures, preventing downtime, and thereby decreasing wasting costs on unnecessary maintenance and labor.
Whether you plan to integrate your energy hardware with sensors and enable IoT connectivity, audit and improve an existing system infrastructure, set up accurate data collection, or develop ML models for predictive maintenance – we've got you covered.
Share the issues you face with the maintenance of your energy systems with our experts, and we will get back to you with an action plan shortly.
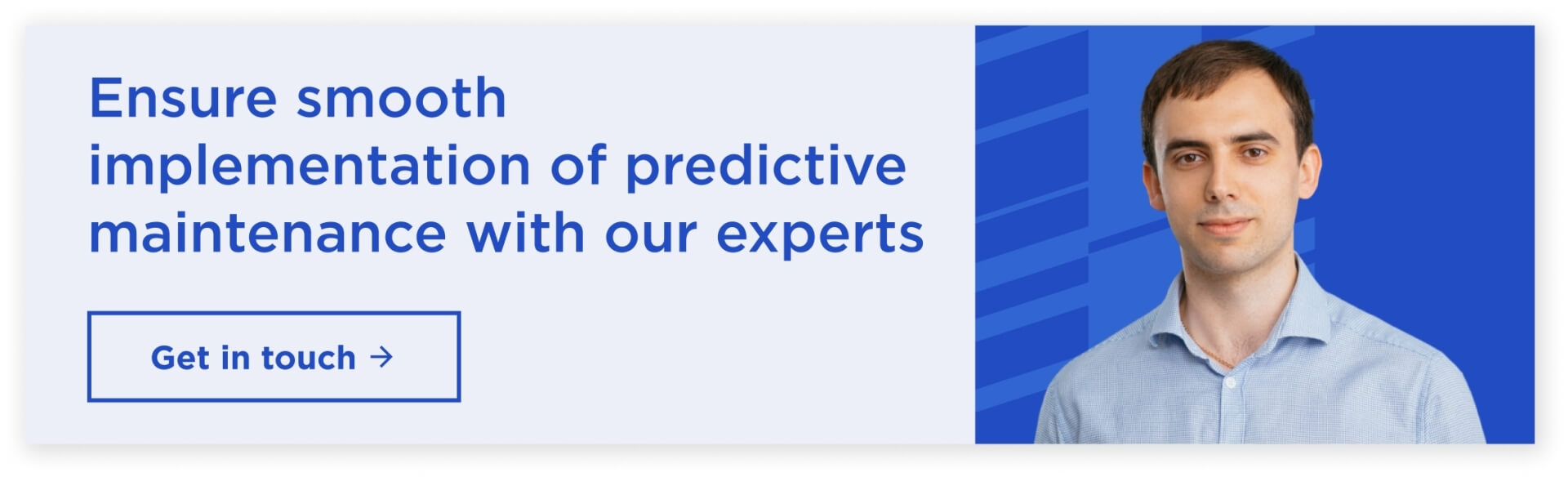
FAQ
Why is predictive maintenance necessary for energy systems?
Predictive maintenance makes the energy system performance more stable and reliable by detecting issues early on and fixing them before they cause any troubles with the system's operation. It also makes maintenance activities more focused on areas where repairs are needed.
How does predictive maintenance optimize costs for energy system maintenance?
Energy system downtime can cause serious consequences for your budget and brand image. Predicting potential system failures ensures you fix them before they stop the system's operation. In addition, there is no need for an onsite maintenance team as you can monitor equipment and environmental conditions remotely and make repairs only when necessary, not by schedule.