Wind turbines operate under unpredictable — and often severe — weather conditions. This makes wind farm maintenance difficult and expensive.
Not only do wind farms lose money when there isn’t enough wind — too much wind can be damaging. A small malfunction, unnoticed under a moderate workload, can cause a system shutdown in heavier wind.
Predictive maintenance is a great way to mitigate this issue. A study by the Electric Power Research Institute found that predictive maintenance techniques can cut annual wind turbine maintenance costs by up to 47 percent. And as turbines collect data about themselves, they’re perfect candidates for predictive maintenance based on artificial intelligence (AI).
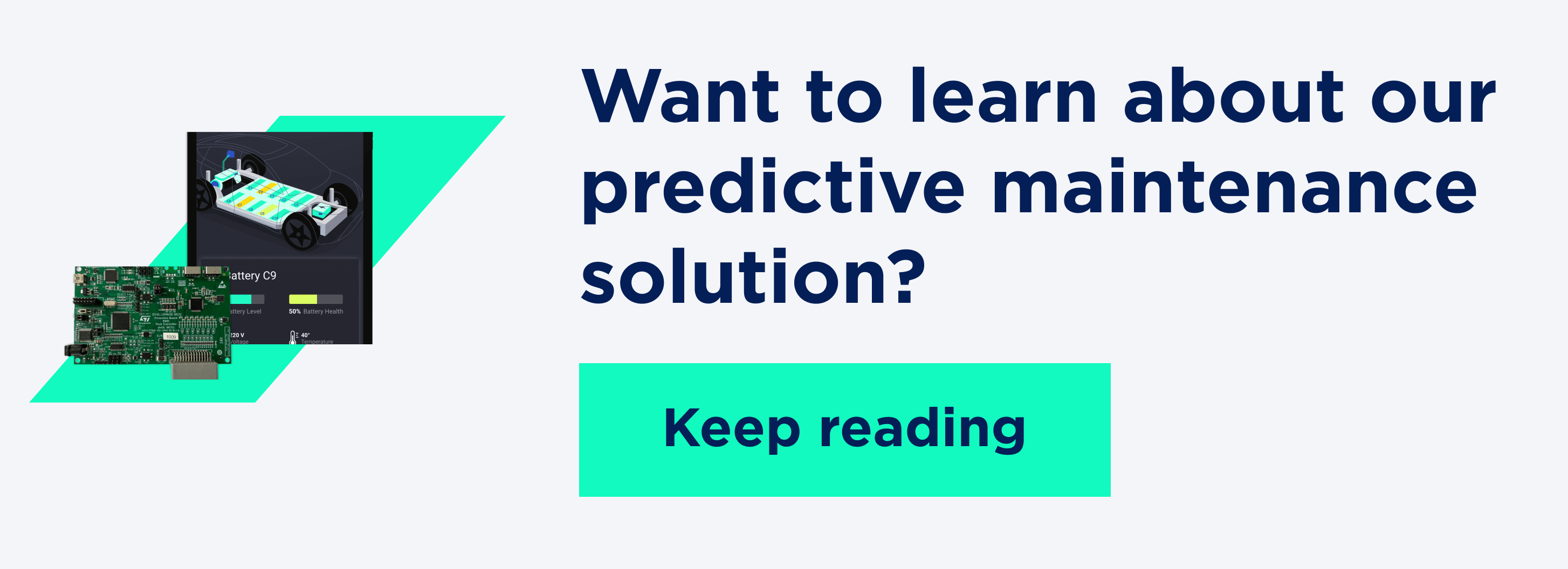
Why use AI for predictive maintenance of wind turbines?
On average 80 meters (262 feet) tall, a modern wind turbine consists of a tubular steel tower, which supports a nacelle and a hub with three blades.
The nacelle is the construction behind the blades. It houses the shaft, gearbox, generator, and supervisory control and data acquisition system (SCADA).
Among other things, the SCADA monitors turbine performance. It usually collects data from over a hundred different sensors:
- Wind data (speed and deviation)
- Performance data (power output, rotor speed, blade pitch)
- Vibration data (tower acceleration, drive train acceleration)
- Temperature data (bearing temperature, gearbox temperature)
This data is key to predictive maintenance of wind turbines.
It takes comparing readings from many different sensors at once to understand the performance of a single turbine. But the sheer size of SCADA data sets makes manual processing impossible. And what’s worse, different readings will mean different things under different environmental conditions.
AI is the solution in cases where there’s too much data for a person to process. An AI system can analyze thousands of data points at once and find connections between them that a person never could.
Let’s look at a specific wind turbine maintenance issue that AI can solve.
Using AI to prevent main bearing lubrication issues
The main bearing connects the hub and the nacelle. If well-lubricated, it can withstand incredible workloads. But without enough lubrication, it can overheat and the turbine will shut down.
Source: Ian Baring Gould, The National Renewable Energy Laboratory
Now let’s imagine that the main bearing lubrication system begins to fail. It’s pumping less lubricant than it should. This makes the main bearing temperature increase steadily. But the change isn't drastic, and the temperature is still in the safe range, so the SCADA doesn’t pick up on anything.
When your technicians turn the turbine to face stronger winds, however, the main bearing overheats, and the turbine stops. While this halt may seem sudden, it’s been a while in the making.
Most existing wind turbine monitoring systems analyze the work of each node and component separately. They make sure that sensor readings do not exceed a safe range of values. But a value can stay in the safe range for a long time until some (not necessarily critical) change in the environment causes it to spike.
The only way to predict the issue in our example is by correlating the amount of lubricant the turbine has been using with the steady rise in the temperature of the main bearing.
One of the major benefits of AI is the ability to pick up on such non-linear connections. As a result, AI systems can find issues before they become emergencies.
A predictive maintenance system with AI can visualize the P-F curve for each turbine component. This curve shows the period of time between the onset of component failure and the moment of actual failure.
With smart notifications, the system can alarm turbine operators when something goes wrong. This will help them plan, schedule, and run maintenance activities before the turbine fails.
The only challenge to building a predictive maintenance system with AI is the way wind turbines collect data. Let’s explore this issue and how you can overcome it.
AI and predictive wind turbine maintenance in real life
Pavlo Tkachenko, a data scientist at Lemberg, has worked on a system for AI-enabled predictive maintenance of wind turbines. His task was to build a system that would prevent turbine failures as opposed to mitigating them.
To build this system, Pavlo had a set of unmarked data on turbine performance throughout the year, represented in five-minute slices. Each slice was a combination of 160+ turbine sensor readings.
After clustering this data, Pavlo cleaned the data set so it contained only data that described normal system behavior. This meant excluding edge cases, removing data that didn’t concern turbine performance, etc.
Still, the fact that this data set contained no representation of undesirable system behavior posed an issue. Because to train an AI system in classification, you need to show it examples of both desirable and undesirable behavior. This way, it will learn to differentiate between good and bad performance of different turbine components.
To work around this issue, Pavlo trained an autoassociative neural network using only the data on desirable behavior in the training set. Trained this way, the neural network could figure out whether data represented desirable behavior even though it was not explicitly shown examples of undesirable behavior. In other words, an AI system trained this way knows what is “good” and can mark anything that doesn’t fit that profile as “bad.”
Even with limited data, Pavlo managed to build a system that predicted turbine failure 48 hours in advance. This provided plenty of time to fix any issues, even for offshore wind farms.
This approach was possible only because the training data set was for an entire year. If it only represented system behavior in summer and autumn, for instance, the AI wouldn’t know whether to mark system behavior in winter as normal.
If you’d like to learn more about collecting data for AI, read our article on how to prepare training data for better AI.
Key takeaways
Predictive maintenance of wind turbines combined with AI can cut wind turbine maintenance costs up to 47 percent.
The main benefit of predictive maintenance systems is their ability to generate a P-F curve. This lets wind farm operators schedule and run maintenance activities as early as 48 hours before a system sustains any major damage.
Let’s work on better wind turbine maintenance systems together
Wind farms are difficult to run, but AI can make it easier. To learn more about AI software development services at Lemberg Solutions, take a look at our case studies.
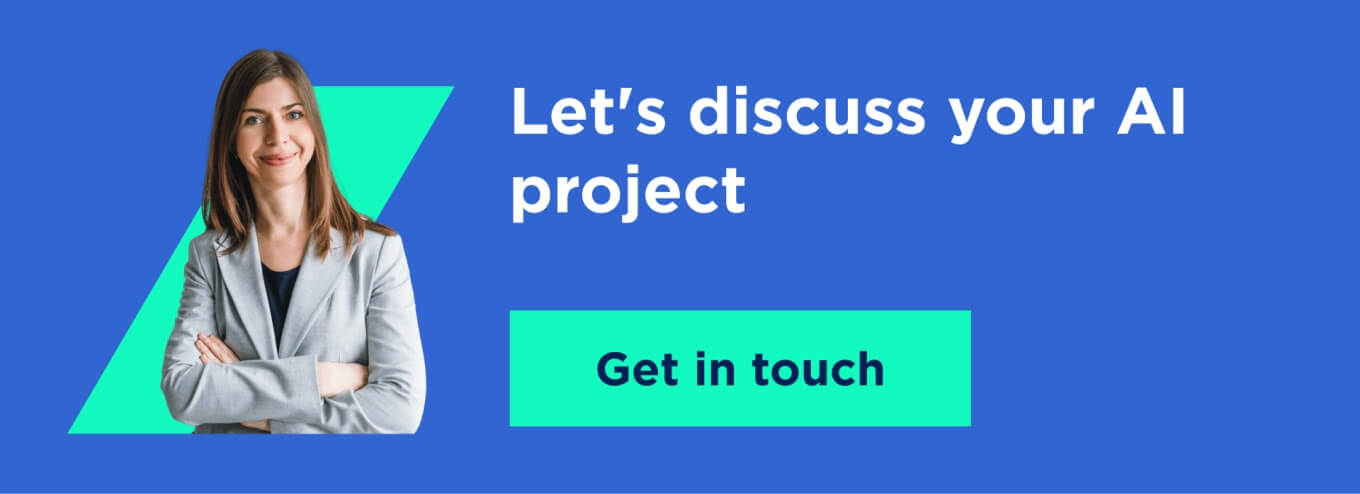