Do you want to integrate AI into your solution to optimize your business but don’t know which type of AI you need? Leverage our data science expertise to figure out what decision to make and gain insights from our introduction to generative AI and predictive AI.
Both of them revolutionize the approach companies take to improve their products, services, and internal operations. Generative AI enables quick and accurate text and image creation. Predictive AI empowers businesses with algorithms that anticipate customer behavior, market fluctuations, and trends.
AI technologies rapidly evolve, and understanding the difference between predictive and generative AI is vital to keeping up with the pace of the market. In this article, we explain how generative and predictive AI can help you grow, describe their benefits, and share practical use cases. Choose AI that meets your needs and start transforming your company.
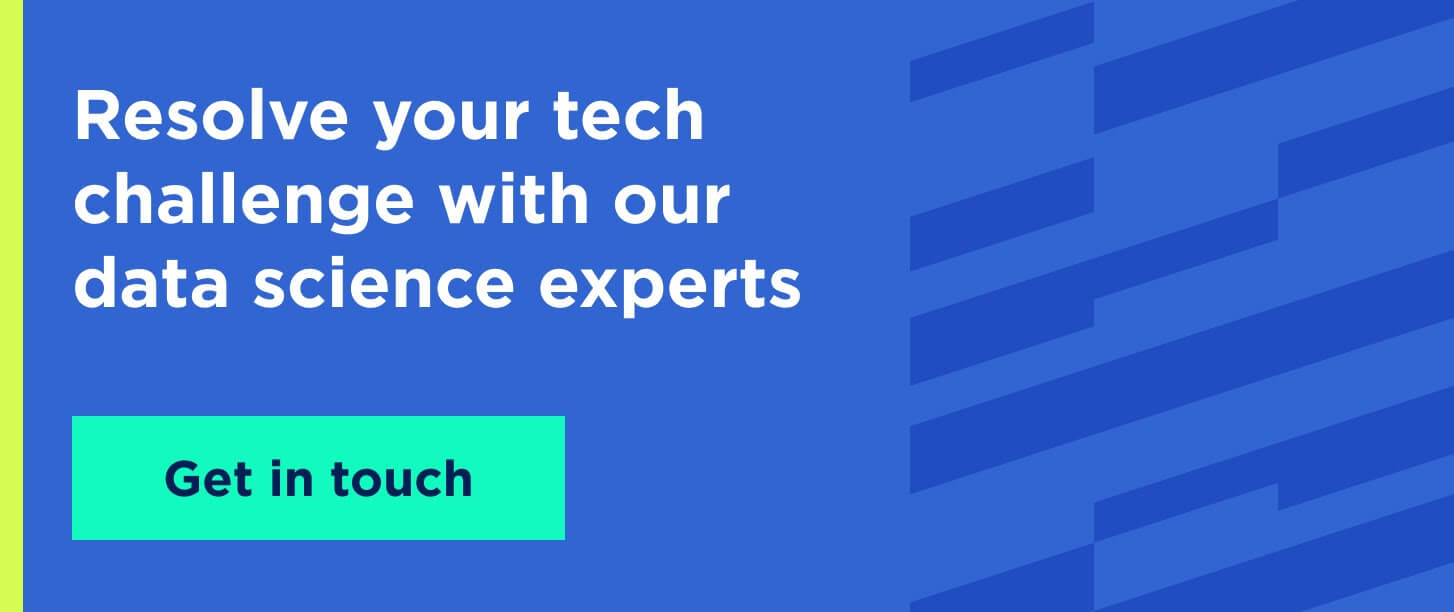
What is Generative AI?
Before we discuss generative AI vs predictive AI examples, let’s figure out the peculiarities of generative AI, how it works, market growth, challenges, advantages, and use cases.
How generative AI works
Generative AI runs on specific algorithms that can analyze and learn data patterns within different kinds of datasets. Unlike traditional AI, genAI can generate new content based on the information, language, context, and patterns provided by data science engineers. You can use generative AI to create text, images, music, and videos that resemble those created by humans. Generative AI models consist of two core parts: a generator and a discriminator. The generator creates new content, while the discriminator estimates the accuracy of the generated content compared to real-life examples. Due to repeated training, the generator learns to develop more realistic results, which leads to the creation of authentic content.
Generative AI market perspectives
Since generative AI is taking on the majority of routine, manual tasks, businesses should prepare for rapid market growth. Such industries as education, legal, IT, and healthcare will be transformed soon. Using natural language processing, GenAI resolves numerous tasks in real time, which attracts various companies globally. Here are more detailed statistics of GenAI's impact on different jobs in 2023:
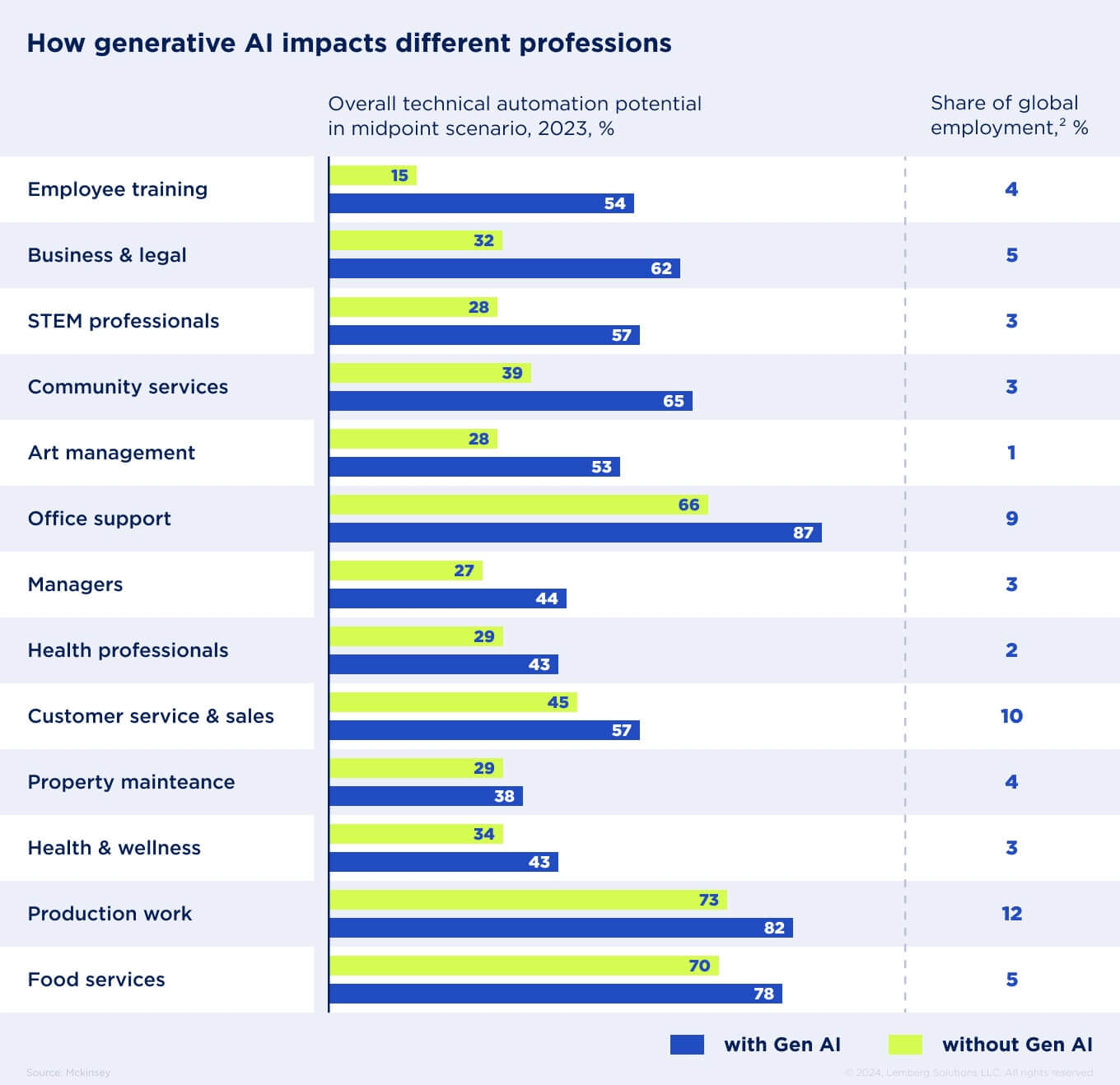
Challenges of implementing generative AI
One of the main generative AI technology integration challenges is the quality and reliability of training datasets. The second challenge stems from the first — inaccurate and irrelevant outputs. Additionally, people are concerned about ethical consequences, such as the creation of deepfakes to spread misinformation with ill intentions. Another challenge is that collecting large amounts of high-quality training data can be difficult or costly. However, despite these challenges, professional data scientists mitigate the risks by providing tech consulting or AI discovery services to conduct the necessary research and help businesses avoid any pitfalls.
Advantages of generative AI
Adopting generative AI may be a complex task; however, the pros outweigh the challenges. Read below to learn how GenAI can help improve your business operations.
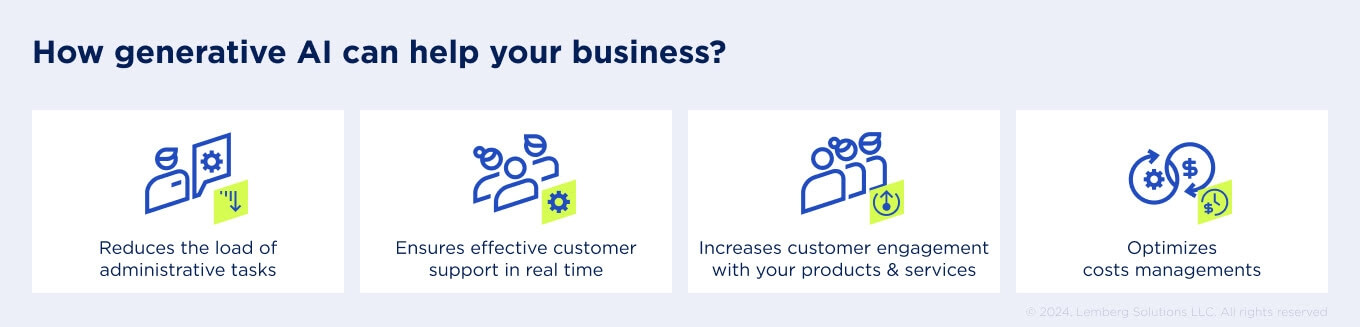
- Reduces the load of administrative tasks
By automating repetitive tasks with GenAI, including report generation, email classification, and document processing, you can significantly reduce the burden on your employees. Your GenAI solution can generate detailed reports based on custom data inputs, so you won’t need to prepare them manually. Moreover, generative AI algorithms can schedule your appointments and help with correspondence, allowing you to focus on higher-level tasks. The algorithms constantly improve based on the current requests and data, ensuring greater accuracy. This way, genAI can increase the company’s productivity.
- Ensures effective customer support in real time
Among other benefits of generative AI, note how it can provide personalized assistance in real time. Chatbots powered by generative AI can interact with customers in a natural way, understanding their sentiments and the context of requests to provide relevant and accurate responses. Such chatbots can handle numerous customer queries simultaneously. Human intervention may be required for the most complex queries. Chatbots with GenAI learn from past interactions, operate 24/7, enhance customer satisfaction, and decrease support costs for companies.
- Increases customer engagement with your products and services
Generative AI offers personalized recommendations to captivate end users and encourage them to purchase relevant products. By analyzing customer behavior and preferences, GenAI algorithms create custom product suggestions that resonate with individual customers. As a result, companies improve their sales and conversion rates. Additionally, you can apply GenAI for product reviews to help customers find exactly what they want. Ecommerce platforms powered with GenAI will stay ahead of the market and boost customer satisfaction.
- Optimizes cost management
Automation of routine operations allows employees to delegate burdensome tasks to GenAI algorithms. This way, companies can optimize resource allocation by distributing tasks between employees and GenAI system. Additionally, you can improve your decision-making with the GenAI, which will lead to making more cost-efficient steps while growing your business.
Generative AI applications
The most common ways how generative AI can be used include chatbots, automated documentation systems, and personal assistants.
Smart chatbots
GenAI-based chatbots can be used across various industries, mainly to enhance customer interactions. For example, in an ecommerce business, such chatbots can provide personalized product recommendations and deal with end-user questions to improve their shopping experience. In the healthcare field, GenAI chatbots can help patients figure out their symptoms, what they mean, and which physician to address. This reduces the amount of work for healthcare professionals. In the finance industry, generative AI-driven chatbots ensure quick and accurate customer support. In the travel industry, smart chatbots can help end users book reservations and choose the perfect travel destination. As you can see, GenAI within chatbots helps deliver personalized and quick customer interactions that boost sales and increase satisfaction with your service.
Documentation processing
You can apply generative AI for document generation, summarization, and sentiment analysis within different industries. Here is how: For law companies, generative AI automates document generation by creating agreements, contracts, and reports based on custom templates and input information. Additionally, GenAI can summarize lengthy legal documents, find valuable key points, and present needed information in a convenient way. By conducting sentiment analysis, GenAI algorithms help ecommerce companies analyze social media posts, end-user feedback, and competitor reviews to optimize their product or service management.
Personal assistants
As a personal assistant within the healthcare industry, GenAI can schedule appointments for patients and physicians, provide needed historical data, and manage patient records. Such assistants can answer patient questions and notify them about taking their medication based on individual health data. In the ecommerce field, personal assistants powered with generative AI can guide customers through online purchases, provide required advice, and inform them about store working hours and locations. As for the finance industry, GenAI assistants can help customers plan their budgets and track expenses.
What is Predictive AI?
To better understand the difference between generative AI and predictive AI, we should dive deeper into the nuances of predictive algorithms, predictive AI market, challenges of implementation, advantages, and use cases.
How predictive AI works
Predictive AI models analyze your data to identify specific trends and patterns, make accurate predictions, and help you take necessary actions ahead of time. Data science engineers train predictive AI using historical data and establishing relationships between variables. Experts label your data so that the algorithms can learn what to look for and how to notify you about any issues or data fluctuations. By implementing predictive AI, you can mitigate risks, predict demand, and more.
Predictive AI market perspectives
In 2020, predictive analytics market was estimated at $5.29 billion, while being projected to grow to $41.52 billion by 2028. This indicates the increasing dependence on predictive analytics across various industries. Predictive analytics is especially helpful for supply chain optimization, finance planning, and customer behavior predictions.
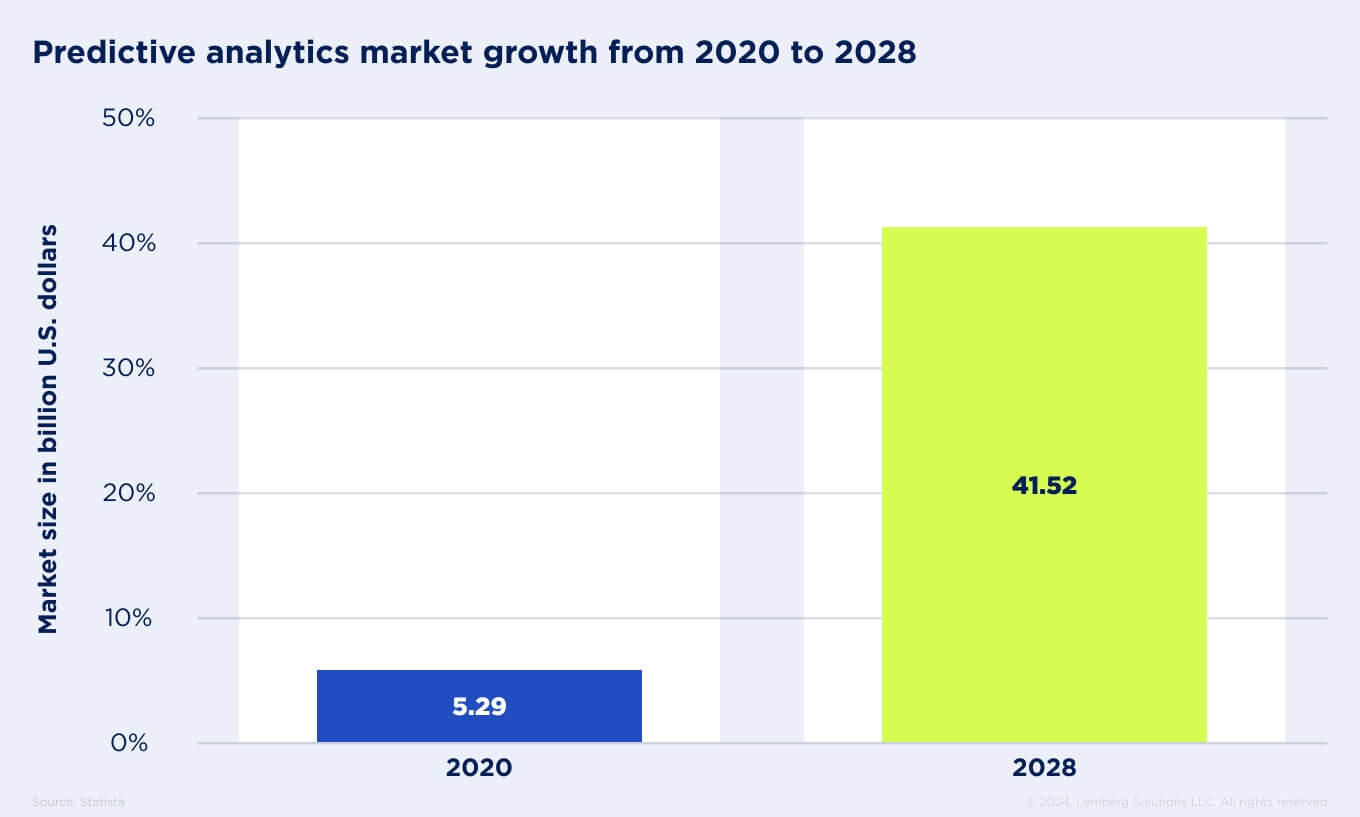
Challenges of implementing predictive AI
Implementing predictive AI requires collecting and preparing high-quality data since the algorithm’s accuracy depends on data relevance and amount. To make sure the data suits all requirements, data science experts clean and preprocess it before further training and labeling. Additionally, data shouldn’t include any biases because the algorithms will make biased predictions, which spoils the decision-making process. Also, the algorithm needs monitoring and updating since you may change your goals and data. However, you can overcome these challenges with the help of our predictive analytics experts.
Advantages of predictive AI
Apart from analyzing patterns, there are such benefits of predictive AI as future trends forecasting, issue prediction, and risk assessment. Read below for more details.

- Anticipates future trends
Predictive AI helps forecast future trends by evaluating large amounts of historical data to detect correlations, new customer preferences, and patterns. You can check market dynamics and identify needed advancements with predictive AI. With early insights from the algorithms, you can stay ahead of the competition and navigate uncertainties with confidence.
- Predicts issues
Predictive AI will forecast potential issues and alert you about any suspicious data patterns and anomalies that imply potential problems. Predictive AI algorithms evaluate various factors to predict the likelihood of specific events or outcomes. Moreover, predictive AI improves its accuracy so you can proactively resolve the challenges and minimize negative impacts.
- Assesses risks
Predictive AI works well for risk assessment since it can evaluate vast amounts of data from internal and external sources to measure potential impact of different events on your business. Predictive AI algorithms can identify anomalies and data patterns inherent to your domain so you can take proactive steps to mitigate potential negative effects. With such early warnings, you can effectively implement targeted risk reduction approach. With predictive AI, you can continuously monitor the market you are interested in and reshape your current operations. Predictive AI ensures that you are always up-to-date with important changes, responding to risks without delay.
Predictive AI applications
There is a difference between types of AI generative vs predictive use cases across various industries. In the healthcare industry, predictive AI systems can assist physicians in analyzing patient data and predicting possible diseases and treatment plans. Patients can also apply a chatbot with a predictive AI engine to evaluate any health risks and take preventive measures. As for the finance industry, predictive AI helps to anticipate any market risks and trends. This way, companies can improve their investment strategies. Ecommerce companies can apply predictive AI to forecast demand and trends so that they can quickly adjust their services and optimize inventory management. Moreover, predictive AI applications include improving your supply chain management.
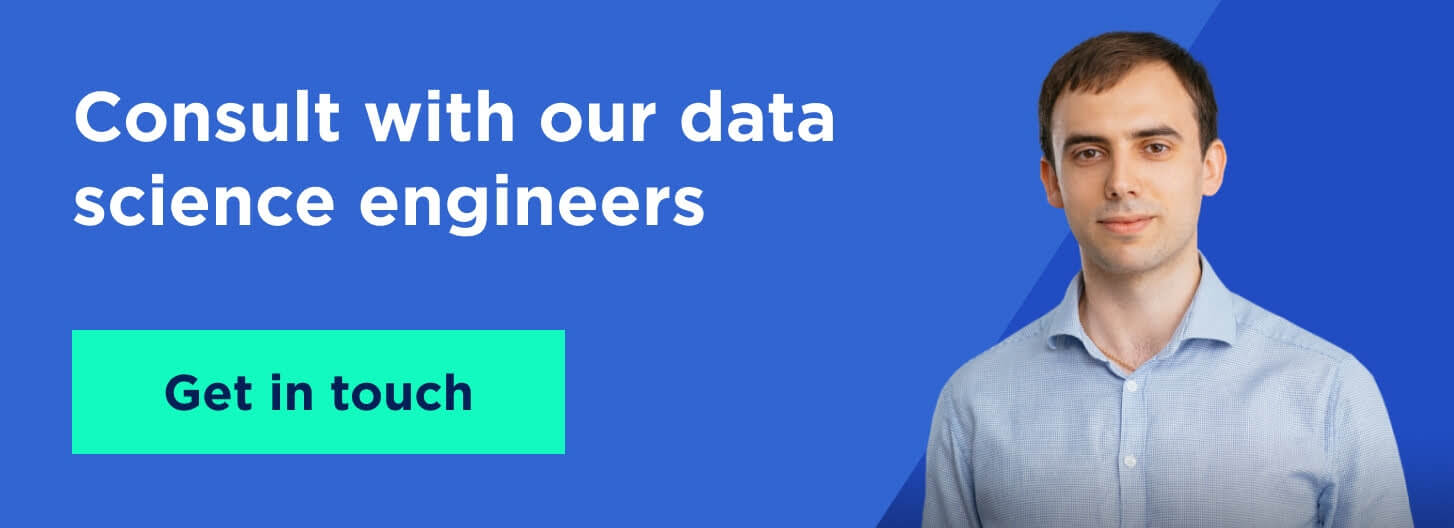
Difference between generative AI vs predictive AI
Generative AI revolves around creating human-like content, including text and images, while predictive AI analyzes specific patterns to make accurate predictions, which are especially useful for sales forecasting and market demand. Learn more in our predictive vs generative AI comparison table.
Characteristic | Generative AI | Predictive AI |
---|---|---|
Business need resolution | Automate routine tasks (e.g., customer support, documentation, summarization, email classification) | Risk assessment and management, issue prediction, market trends forecasting, demand anticipation |
Use cases | Smart chatbots, documentation processing system, AI-based personal assistant, recommendation engine | Predictive analytics system, forecasting chatbot, market analysis and prediction software |
Challenges | Dataset quality and reliability, output accuracy | Dataset quality and reliability, output accuracy |
Complexity level | Requires a large amount of high-quality data, depends on the domain and goal, results should be human-like, relevant, and realistic | Requires large amount of high-quality data, depends on the domain and goal, statistical analysis |
Tech stack | Python, PyTorch, AWS, Azure, ChatGPT, scikit-learn, Milvus, spaCy, Cohere | Python, NumPy, TensorFlow, Keras, MATLAB, Pandas, AWS, Azure |
Takeaway
If you’ve ever wondered what is generative AI vs predictive AI difference and how you can leverage any of these technologies in your business, we are here for you. In case you have any questions left after reading this article, and you need a consultation with professional data science experts — contact us.